Even compared to a year ago, you’re probably seeing more AI applications in your day to day life. You might be seeing AI assistants cropping up in your email inboxes offering to help summarize messages and compose text. Your phone might be offering new AI-powered capabilities, and depending on how well they work life is either a little bit easier or a little bit more annoying.
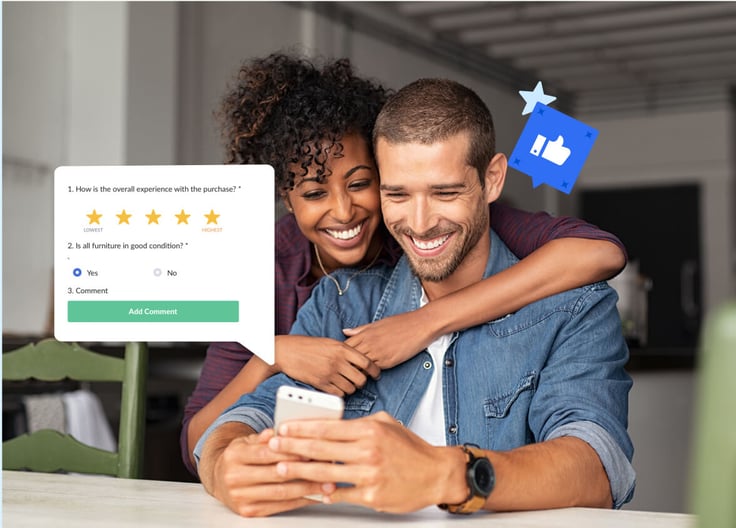
At this stage, it feels more like an evolution than a revolution. ChatGPT hasn’t taken over the world yet, and human delivery drivers still haven’t been phased out in favor of autonomous vehicles with sophisticated computer vision capabilities—but AI has been making an impact in the world of logistics for longer than most people imagine. In fact, many solutions that have been on the market for years have been leveraging AI and machine learning (a subfield of AI) to improve performance and boost users’ capabilities for many years.
As AI research progresses and the technology becomes more and more sophisticated, its potential application in logistics in general (and last mile logistics in particular) is only going to grow. To lay the groundwork for future applications, businesses that are interested in the power of these kinds of advances already need to be thinking about undergoing digital transformations and future-proofing their technology stacks.
Make no mistake: the uses of AI that are already available to delivery organizations are already powerful enough to impact efficiency and boost profitability. But these solutions also have the advantage of positioning their users to more easily take advantage of new applications of AI in logistics as they crop up.
1. Route Optimization
At heart, AI and machine learning are all about data. To “teach” programs to do certain things as well as—or better than—their human counterparts, they usually need to be trained on large datasets. When it comes to route optimization, some of the most crucial data out there is about travel and service times for traveling from point to point along a route. Given huge amounts of data on previous deliveries, previous traffic patterns in a given region, and more, machine learning algorithms can predict ETAs with greater accuracy than a human planner could ever hope to achieve.
This might not sound like an earth-shattering use of AI—but in point of fact it can underpin radically smarter, faster, and more cost-effective route optimization processes. How long it’ll take to complete a given delivery after you’ve wrapped up at the previous stop determines how many deliveries you’ll be able to make in a day, and it also determines whether you’ll be able to meet time requests made by your customers. In other words, having accurate ETA predictions is the foundation of any route plan. Without them, you don’t just risk late deliveries, you also risk potentially scheduling more deliveries than your capacity allows for, which can have huge downstream impacts.
In this way, AI-powered route optimization enables you to generate more accurate routes much more quickly and easily. And this technology isn’t a hypothetical—it’s an application of AI in logistics that’s making an impact on businesses' last mile operations right now.
2. Customer Experience
A chatbot that leverages natural language processing (the kind of technology that ChatGPT and similar tools rely on) can help boost efficiency and streamline customer experience in the last mile—if you’re deliberate about the way you deploy it. For starters, the technology needs to really work; your customers are going to be upset if the AI hallucinations and promises something that your actual operations can’t deliver. By the same token, if it can’t resolve their inquiries at all, they’re going to get frustrated, call you up, and take out their frustration on the sales or customer service team.
When it comes to leveraging AI for customer experience improvements, it’s crucial to think about the ways that technology can and can’t make life easier for your customers. Some platforms will try to sell you on the idea that your customers would rather interact with an AI-powered chatbot than a human being—but that simply isn’t true. The current state of AI technology can make chatbots a valuable tool, but they have to be deployed in a way that augments the humans on your team rather than trying—in vain—to replace them.
What might that look like in practice? Probably not too different from the chatbot technology you’re picturing—the trick will be to make sure that it’s integrated into a larger customer experience program.
Customers might be receiving regular updates from your team about delivery schedules, ETAs, and more, and they might have the option of reaching directly out to the team with any questions. Here, AI can work as a front line for getting the customer an answer more quickly. Rather than waiting on a human to respond, the customer can ask their question and either receive a response or be routed to a human being who can help with the answer. This reduces the volume of customer inquiries that members of your team have to deal with, giving them more time to help resolve situations that really require human touch.
AI-powered functionality can also help suggest responses in two-way communication channels between customers and dispatchers or drivers, speeding up responses and streamlining the process. Again, none of these are a substitute for clear, consistent communication or actual human intervention when needed—but they can help your customers feel like they’re being taken care of while making sure you’re able to focus on what matters.
3. Predictive Insights
One thing that’s probably clear by now is that AI and machine learning have practical applications in predicting outcomes based on large caches of logistics data. And those applications go beyond predicting ETAs and efficient territory organizations. For instance, some businesses might use AI to predict the likelihood of customer order quantities in advance. Elsewhere in the supply chain, you’re starting to see technology for predicting favorable freight prices (basically the supply chain equivalent of the Google Flights functionality that tells you when prices are low).
This is another area where data is king. When your operations are fully digitized, logistics processes—including last mile logistics—can generate a lot of operational data, and AI can help you make the most of it. By using past performance to inform your expectations for the future across a number of different functions, you can create smarter, more efficient plans while effectively working to stave off disruptions.
4. Address Resolution
Though it’s absolutely not the sexiest problem being worked on, normalizing addresses can actually be a serious challenge for some delivery organizations. To generate delivery routes and actually execute on them, you need addresses that are accurate and formatted in a consistent way—something you can’t necessarily count on when buyers are inputting their own delivery information. Without a means of resolving addresses, it’s difficult to ensure that your drivers are actually delivering to the right place at the right time.
Here, AI is already quietly making an impact. By leveraging machine learning trained on innumerable addresses and delivery locations, last mile logistics operators can speed up and simplify address resolution, resulting in improved routes, more empowered drivers, and happier customers.
5. Future Proofing for New Applications of AI in Logistics
We’ve discussed just a handful of the applications of AI in logistics that are already making life easier for dispatchers, managers, and drivers across the delivery sector—but there’s no doubt that more innovations are coming down the pike in the future. At least for the time being, new uses of AI in the last mile are likely to involve incremental advances, but they still have the power to impact logistics businesses in ways that we probably haven’t even considered yet.
But which businesses are actually going to reap the rewards as new AI-powered technologies make themselves available? It’s going to be the ones that laid the groundwork now by prioritizing digital transformation and adopting existing AI-powered solutions. Simply put, by integrating AI and machine learning into existing processes now, you can pave the way for much easier implementation of newer technologies. AI, in other words, can act as a way to future proof your supply chain technology stack—in addition to conferring all the immediate benefits we discussed above—for businesses that are willing to prioritize it now.